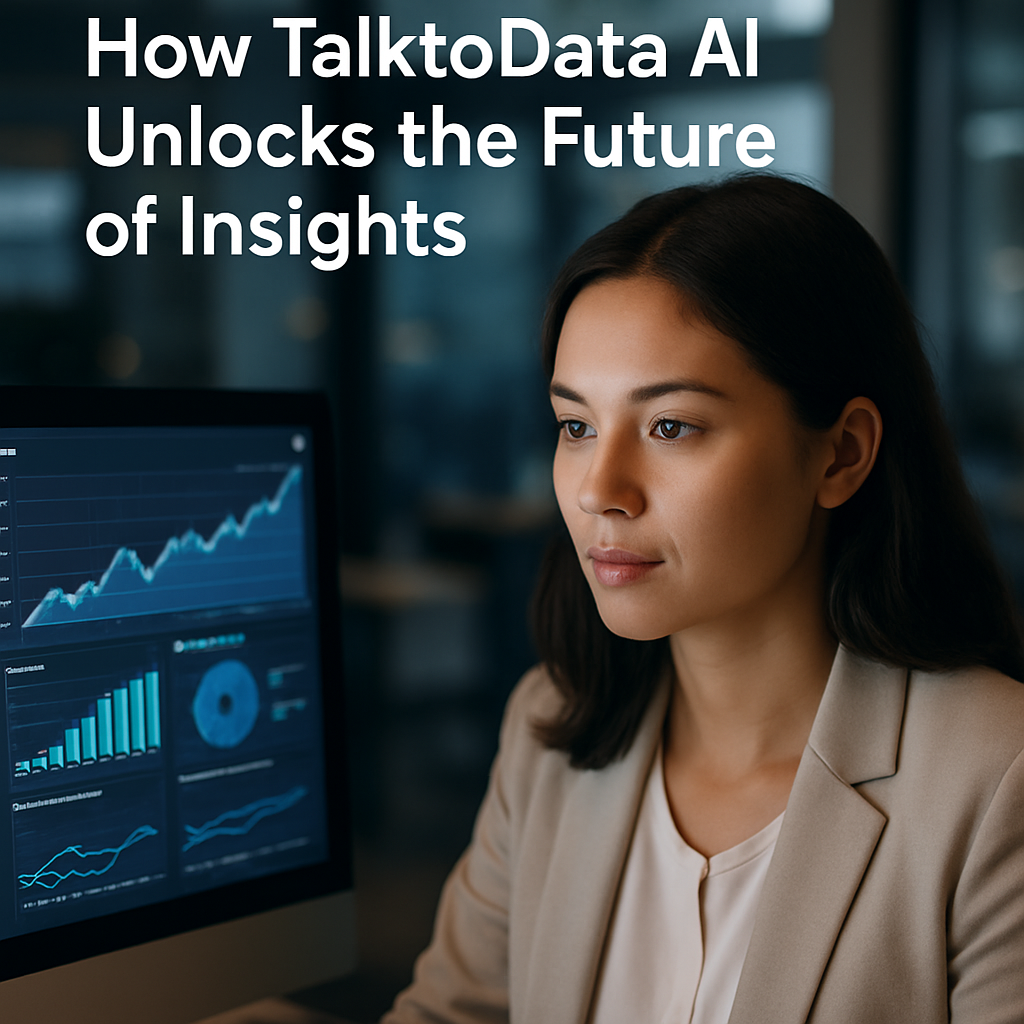
Retail Analytics for Inventory Optimization
Introduction:
Imagine a bustling retail store during the holiday season. Shelves quickly empty of popular items, while less-desired products pile up unsold. What if there was a more systematic way to anticipate demand, avoid stock-outs, and reduce overstocking? That’s where Retail Analytics for Inventory Optimization steps in. By harnessing data insights, retailers can align product availability with customer needs, streamline operations, and ultimately drive higher profits.
Retail analytics brings a wealth of information to the table, from real-time sales tracking to predictive modeling. Effective inventory optimization relies on examining historical transactions, analyzing seasonality trends, and understanding customer buying behaviors. Not only does this approach result in fewer missed opportunities and lost sales, but it also mitigates waste, lowers holding costs, and improves overall supply chain strategy.
In this blog post, we will explore how data-driven insights can transform inventory management practices across various retail sectors. We’ll dive into the types of data retailers need, the technology solutions that enable analytics, real-world use cases, and how different industries can apply these principles. By the end, you’ll see how a proactive stance on inventory management drives growth and keeps customers satisfied. Now, let’s take a closer look at why this matters so much in today’s fast-paced, consumer-centric marketplace.
1. Understanding the Power of Retail Analytics
At its core, retail analytics is the process of using data to decipher patterns and behaviors within a retail environment. From foot traffic counts to customer loyalty data, every piece of information can provide actionable insights to optimize inventory levels. But why is this so important? In an era of digital transformation, consumers expect products to be available whenever and wherever they want them. Falling short of these expectations can lead to lost sales and damaged brand reputation.
Through Retail Analytics for Inventory Optimization, businesses gain the ability to forecast demand accurately. This involves sifting through historical sales figures, seasonality data, economic indicators, and even external factors such as local events or weather patterns. By combining these data points, retailers can create predictive models that help determine when to restock certain products and how to phase out items nearing the end of their lifecycle.
Consider a mid-sized clothing retailer struggling to keep up with changes in fashion trends. By embracing analytical tools, the retailer can swiftly identify which styles and sizes are in highest demand. This enables the store to reorder the right mix of products at the right times, limiting markdowns on slow-moving stock. Furthermore, analytics can guide retailers on where to allocate inventory across multiple locations, ensuring every branch carries items that resonate with its local customer base. The result? Leaner operations, less waste, and a more satisfying experience for both managers and shoppers. When harnessed correctly, retail analytics truly empowers businesses to become more adaptable and competitive.
2. Key Data Points for Effective Inventory Optimization
Transforming raw information into strategic action requires focus on the right data points. First and foremost, point-of-sale (POS) data is invaluable. Each transaction reveals how often a product sells, at what price, and in which promotional context. POS data, layered with seasonal trends such as back-to-school or holiday rush periods, can highlight peak demand times. By centralizing this information in a unified platform, retailers can identify emerging patterns more quickly and make informed decisions about inventory replenishment.
Additionally, warehouse and logistics data play a key role in Retail Analytics for Inventory Optimization. Understanding shipping schedules, receiving times, and distribution center capacity helps streamline the flow of goods. Data-driven insights into supplier performance also ensure that replenishment schedules remain reliable, minimizing the risk of stockouts. Matching your forecasting models with projected delivery timelines creates a solid foundation for effective inventory planning.
External sources, such as market trends and competitor pricing, enrich your existing datasets. For instance, a sporting goods retailer monitoring new product launches by competitors may adjust its own inventory strategy to capitalize on market excitement or fill in gaps that rival products leave unaddressed. Gathering customer sentiment data via social media platforms and reviews can further refine your approach, helping you discern which niche products are making waves in online communities. In essence, capturing the right data and integrating it into a holistic view of your operations can act as the catalyst for accurate demand forecasting and lean inventory strategies.
3. Real-World Use Cases and Success Stories
While theoretical knowledge is important, real-world applications demonstrate the transformative power of Retail Analytics for Inventory Optimization. One notable example is a global e-commerce giant that uses predictive analytics to gauge future demand. By analyzing countless data points—from individual purchasing histories to keyword search trends—its supply chain team can accurately anticipate spikes in product interest. This approach allows them to fine-tune inventory levels, reduce shipping times, and keep customers coming back for more.
Another illustrative case comes from a regional grocery chain that adopted advanced analytics to enhance its perishable goods management. Historically, the retailer faced significant spoilage and was unable to predict the right quantity of fresh produce to stock. After implementing machine learning models that factored in local weather forecasts, holiday events, and promotional activities, the chain significantly cut waste while still maintaining high in-store availability. This not only curbed losses but also elevated the shopping experience with consistently fresh offerings.
Smaller boutique stores have also leveraged analytics solutions. Take, for example, a niche bookstore that began tracking author signings and local reading events. By estimating demand surges tied to these events, the store accurately scaled its inventory for upcoming signings. This prevented undersupply, improved customer satisfaction, and contributed to a sharper competitive edge in the local community. In each of these use cases, the underlying message is clear: with the right analytics capabilities in place, retailers can elevate their strategies from reactive guesswork to proactive, data-informed planning.
4. Industry-Specific Insights for Inventory Management
The beauty of Retail Analytics for Inventory Optimization lies in its adaptability to different sectors. In the fast-fashion industry, for instance, product turnover is rapid and success hinges on getting trends right before they fizzle out. Analytics-driven forecasting enables fast-fashion retailers to restock hot items efficiently while phasing out slower-moving lines. This quick response leads to fewer markdowns and improved profitability.
Meanwhile, electronics retailers often deal with product life cycles that are influenced by technological innovations. A sudden smartphone upgrade announcement can make current models less appealing almost overnight. Analytics tools can help predict these shifts in demand and adjust inventory accordingly, allowing retailers to clear old stock before it becomes obsolete. Even in home improvement stores, demand can soar for certain items after weather-related events—think of the surge in demand for snow shovels ahead of a blizzard or portable generators during hurricane season. Retailers armed with timely data can respond proactively, positioning the right products in the right areas of their stores.
Industry-specific insights also extend into areas like luxury goods, pet supplies, and auto parts. Each sector has its own set of unique metrics and nuances—it’s not always about speed of turnover, but also product reliability, brand perception, and regional preferences. By tailoring analytical models to these industry characteristics, retailers can anticipate customer needs more effectively. Furthermore, internal links to dedicated resources or external references like industry reports from Deloitte or the Harvard Business Review can offer additional perspectives on sector-driven best practices. With an industry-specific approach, businesses unlock a higher return on their analytics investments, as each insight speaks directly to their operational realities.
Conclusion
Retailers that embrace analytics as a cornerstone of inventory management stand poised to thrive in a hyper-competitive landscape. By focusing on accurate demand forecasting, strategic stock allocation, and data-driven insights, businesses elevate themselves ahead of the competition—where customer satisfaction and profit margins align more seamlessly. Whether you’re a start-up boutique or a multinational retail operation, the power of Retail Analytics for Inventory Optimization can help you conquer the constantly shifting demands of the marketplace.
As we’ve explored, the key lies in collecting the right data, leveraging advanced technologies, and applying industry-specific insights that resonate with your unique business model. The end result is a leaner, more responsive supply chain with minimized waste, reduced costs, and better service all around. Ready to get started? Ask yourself: is your current inventory management approach keeping pace with modern retail challenges, or could a data-driven strategy make all the difference? We invite you to share your thoughts or personal experiences on bringing analytics into your inventory processes. There’s never been a better time to adopt a forward-thinking mindset that puts customers—and data—at the heart of every decision.