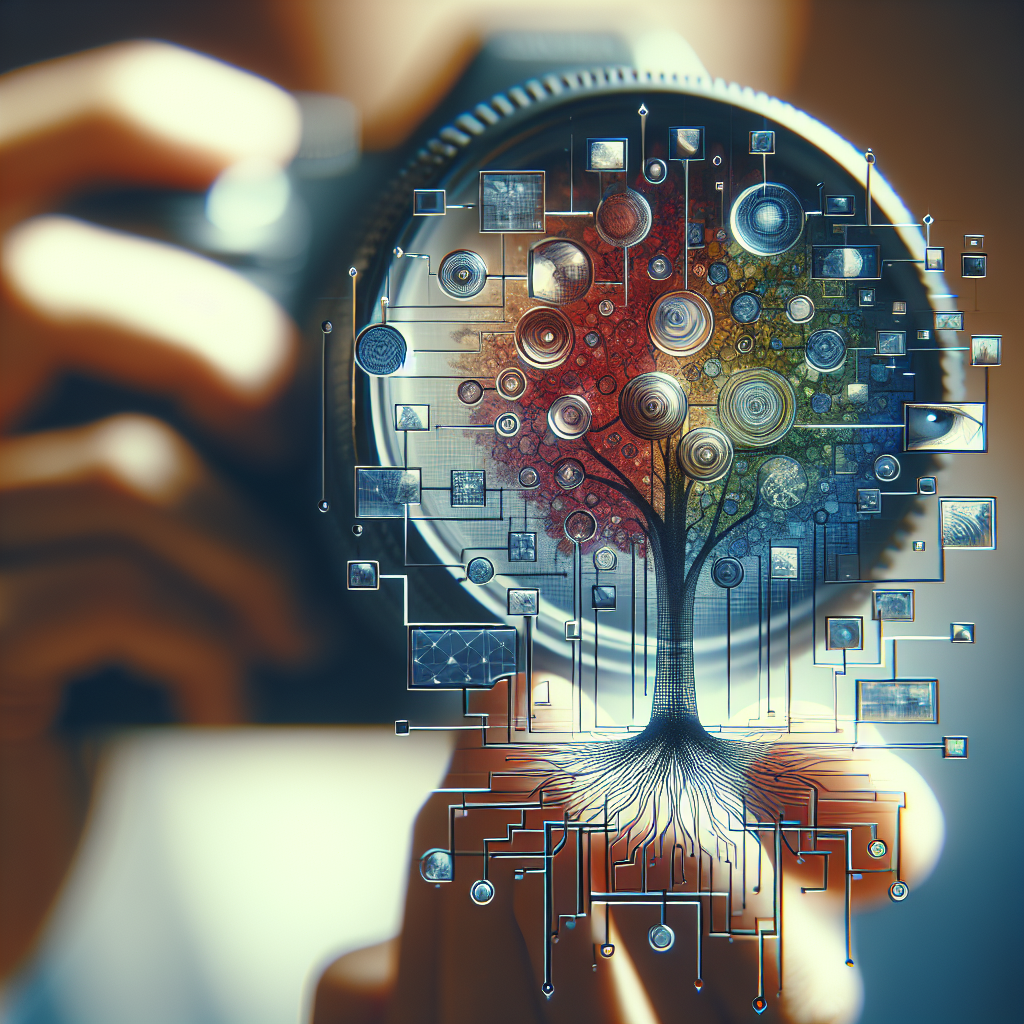
Real-Time and Edge Analytics
Introduction:
Speed and agility are becoming non-negotiable in today’s fast-paced digital landscape. From smart homes that predict energy usage patterns to autonomous vehicles that detect real-time road conditions, data-driven insights have never been more critical. But is simply collecting data enough to keep up with the demands of modern business and society? That’s where the power of real-time analytics and edge computing comes into play.
Real-time analytics refers to processing and analyzing data as soon as it is generated, enabling instantaneous or near-instantaneous actions. Meanwhile, edge computing moves computation and data storage closer to the source of data creation, cutting down on latency and reliance on centralized systems. This duo not only helps organizations make faster decisions, but also addresses the unique challenges posed by streaming data in scenarios where advanced analytics must happen on the fly.
In this post, we’ll explore the significance of real-time analytics and consider how edge computing empowers industries to process massive volumes of streaming data efficiently. We’ll also delve into practical use cases that exemplify their combined potential. Whether you are an engineer responsible for building next-gen IoT ecosystems or a decision-maker seeking a competitive edge, understanding these concepts and their potential applications is essential. Let’s dive in and discover how real-time analytics and edge computing might just redefine the future of data-driven innovation.
Section 1: Understanding the Importance of Real-Time Analytics
Real-time analytics is the process of collecting, processing, and interpreting data as it is generated or received. Imagine receiving actionable insights within seconds of a transaction, instead of wading through hours or days of backlogged data. The implications are enormous: businesses can quickly identify bottlenecks in production lines, financial institutions can spot fraudulent activity before significant damage occurs, and healthcare providers can respond to critical patient data instantly. This speed of response can mean the difference between capitalizing on an opportunity or missing it entirely.
One might ask, “Why now?” Well, the explosion of connected devices continues to generate unprecedented volumes of streaming data every second. Social media updates, e-commerce transactions, and sensor readings in IoT networks all contribute to data streams that are too large and complex to gracefully manage with traditional batch-processing approaches. Earlier, organizations relied on daily or weekly reports to guide decisions. Today, the window of opportunity to act on insights is shrinking, and real-time analytics has become the new standard for staying competitive.
A compelling example can be found in online retail, where e-commerce platforms monitor browsing habits, cart abandonments, and purchasing patterns in real time. Through predictive modeling and instantaneous analysis, these platforms offer personalized product recommendations, which boost sales and improve customer satisfaction. Even a single second’s delay can affect a user’s experience, underscoring why real-time analytics is so crucial.
Beyond e-commerce, fields such as finance, pharmaceuticals, and transportation all benefit from immediate data analysis. Banks that leverage real-time analytics can flag suspicious transactions instantaneously, reducing risks associated with fraud. Meanwhile, logistics companies can adapt delivery routes on the fly if traffic conditions change. These scenarios highlight the transformative potential of real-time analytics in a data-driven world.
Section 2: Exploring Edge Computing for Faster Decision-Making
Edge computing has emerged as a powerful solution to the challenges posed by massive data generation and the need for lightning-fast insights. Instead of channeling every single bit of information to a centralized data center or cloud for processing, edge computing enables computation to be performed at or near the source of data creation. This approach significantly reduces latency because data doesn’t have to traverse long distances before action can be taken. And in time-sensitive environments—think autonomous vehicles or robotic manufacturing—every millisecond counts.
Consider the automotive industry, particularly self-driving cars. Sensors embedded in these vehicles capture streaming data continuously: traffic signals, proximity to other cars, road quality, weather conditions, and more. The sheer volume of this input is astonishing. But waiting for a round trip to a distant server before making a decision could be catastrophic. With edge computing, essential decisions happen on-board, ensuring the vehicle can react almost instantly to changing road conditions.
Edge computing also helps address issues around connectivity and bandwidth constraints. In remote or rural areas, network connectivity might be unstable or expensive. By processing data locally on edge devices, system performance and reliability are maintained even if connectivity to the central cloud is temporarily lost. For instance, a wind farm in a remote location can use edge devices to monitor turbine performance, detect anomalies, and initiate preventive maintenance without relying on constant communication with a distant cloud server.
Furthermore, edge computing often goes hand in hand with enhanced security measures. Where sensitive information is concerned—medical records, confidential industrial processes, or personal user data—keeping analysis closer to the data source reduces the risk of exposure during transmission. Additionally, since analytics occur locally, the risk of data breaches in transit diminishes. As real-time analytics systems continue to evolve, we can expect even more adoption of edge computing architecture to keep businesses agile and efficient, regardless of where data is generated.
Section 3: Capitalizing on Streaming Data
Streaming data is the continuous flow of real-time information from various sources, such as sensors, networks, and online channels. This relentless stream offers organizations a dynamic, up-to-the-second view of operations and user behaviors. But harnessing streaming data effectively requires intelligently designed systems capable of filtering, enriching, and analyzing information without bottlenecks. That’s why real-time analytics and edge computing form a perfect duo to extract immediate value from these flows.
Take social media platforms as a prime example. Millions—sometimes billions—of posts are created every day, forming a dynamic tapestry of user discussions, trending topics, and breaking news. By applying real-time analytics to this streaming data, businesses and organizations can gauge public sentiment, respond to emerging crises, or capitalize on viral trends. This insight is invaluable for tailoring digital marketing campaigns, optimizing user engagement, and quickly adjusting brand messaging.
In an industrial context, consider the oil and gas sector, where pipelines are often located in remote areas. Sensors constantly feed vital operational data—pressure levels, temperature, flow rates—to central supervisory systems. Real-time analytics running on edge computing nodes can detect anomalies, such as leaks or dangerously fluctuating pressure, and instantly trigger alerts. This enables timely corrective actions that minimize environmental damage and operational losses.
The common thread across these scenarios is the marriage of continuous data flow with immediate processing power. Not only does this reduce the downtime associated with data collection and batch reports, but it also opens up new opportunities for real-time engagement and strategic pivots. As technology advances, expect streaming data to be at the forefront of product innovation, customer engagement, and operational enhancements—made possible through a blend of real-time analytics and edge computing solutions.
Section 4: Real-World Use Cases and Success Stories
From healthcare to retail to manufacturing, organizations are reaping tangible benefits by integrating real-time analytics with edge computing. In healthcare, for instance, wearables and medical IoT devices—such as smart insulin pumps and heart monitors—constantly transmit patient data. Real-time analysis on these edge devices can detect abnormalities and immediately alert medical professionals, dramatically improving patient outcomes. In such time-sensitive scenarios, even a brief delay can be detrimental, which underscores the life-saving potential of these technologies.
In retail, some supermarkets are now using camera-based sensors to track foot traffic in different aisles. By applying real-time analytics at the edge, store managers can optimize checkout lines, restock shelves more efficiently, and even adjust in-store promotions on digital signage to reflect changing customer interests. The result is a seamless shopping experience that boosts both customer satisfaction and revenue.
Meanwhile, in the realm of entertainment, major streaming platforms process billions of data points—user preferences, engagement times, completion rates, and more. By employing real-time analytics, these platforms can deliver hyper-personalized content recommendations, thereby keeping viewers engaged. Edge computing plays a role here as well, particularly for local caching to smooth out content delivery and minimize buffering.
As these examples illustrate, the power of real-time analytics and edge computing is not confined to futuristic labs; it’s already at work in our daily routines. Whether it’s optimizing the global supply chain or helping farmers monitor crop conditions from a distance, these technologies have broad, real-world applications. Countless businesses are investing heavily in these infrastructures, and with good reason. The return on investment often comes in the form of operational efficiencies, reduced costs, improved customer satisfaction, and even new revenue streams. And as networks evolve—5G, satellite internet, and beyond—the capabilities of edge computing and real-time analytics will expand tremendously.
Conclusion
Real-time analytics and edge computing are transforming how organizations handle and leverage streaming data. By enabling instant insights where they are needed most—often right at the source—these technologies empower quick decision-making, lower latency, and more resilient systems. This shift from centralized to decentralized data processing is especially valuable in industries that prize agility and rapid response, from critical healthcare scenarios to high-stakes financial transactions.
As you plan your next data strategy, consider how real-time analytics and edge computing fit into your broader roadmap. Balancing both is key to delivering meaningful outcomes: real-time analytics ensures no window of opportunity is missed, while edge computing removes many of the technical barriers that slow data flow. Together, they offer a way to stay innovative, reduce costs, and meet evolving customer expectations.
What possibilities do you see for these technologies in your own organization or daily life? We’d love to hear your thoughts. Feel free to share your perspective, ask questions, or offer insights in the comments below. And if you enjoyed this post, don’t forget to share it with your network. Let’s keep the conversation going and explore how real-time analytics and edge computing can shape an even more connected, efficient future.