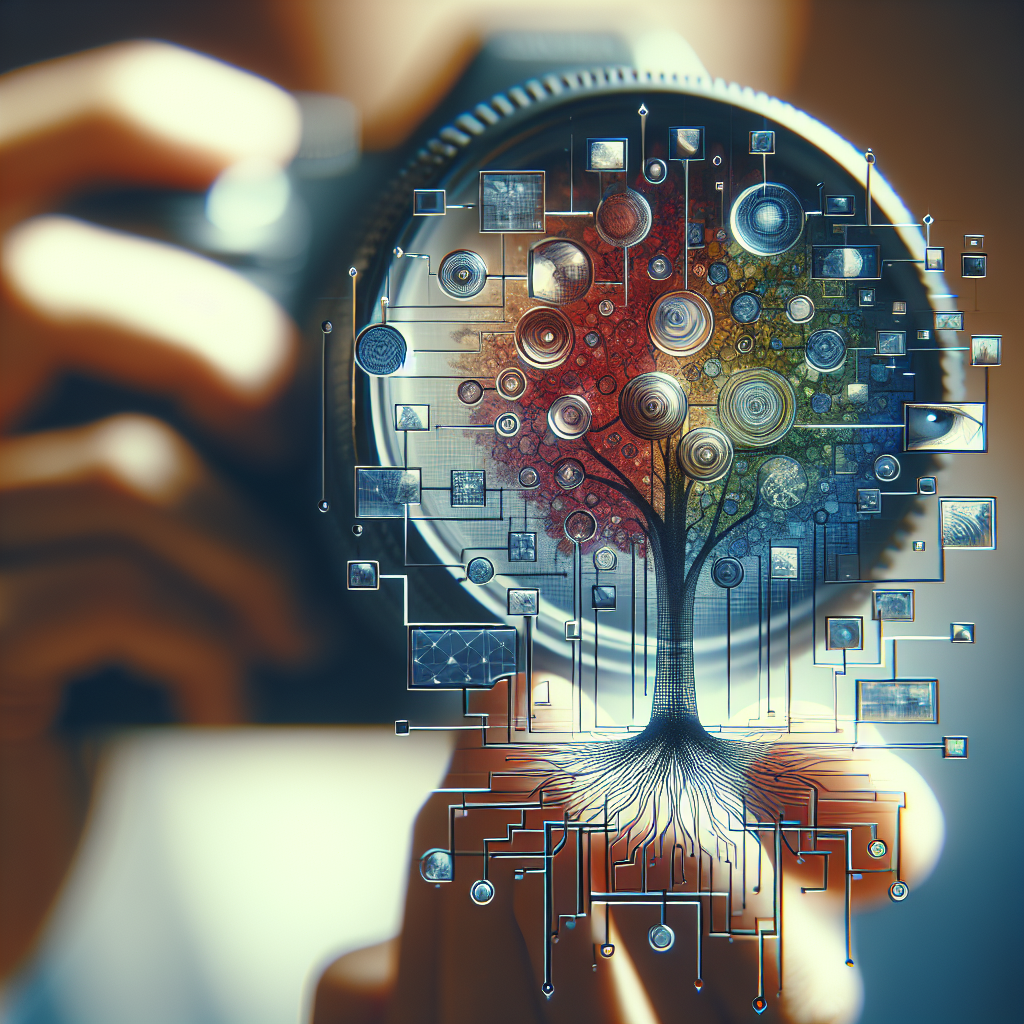
Pharmaceutical Data Analytics: Optimizing R&D
Introduction:
Imagine if groundbreaking medicines could reach patients at lightning speed, saving lives while also driving down costs. Does that sound too good to be true? Modern pharmaceutical data analytics is making this vision one step closer to reality by optimizing every phase of the drug research and development (R&D) lifecycle. From early-stage discovery to post-market surveillance, data-driven insights allow researchers to predict outcomes and spot inefficiencies, accelerating breakthroughs that once took decades to unfold.
In a world where billions of dollars are poured into R&D, the need to make every cent count urges pharmaceutical companies to adopt more agile solutions. Data analytics tools are helping them uncover new potential in existing drug pipelines, identify patterns in clinical trial results, and foresee potential adverse effects before they become widespread issues. With cutting-edge technology at their disposal, pharma teams are making more informed decisions—faster and at a lower cost.
But the promise of analytics goes beyond just cost-savings. It also transforms how these organizations interact with regulators, healthcare providers, and even patients. By establishing continuous feedback loops, pharmaceutical companies can refine products and address safety concerns in real time. In this blog post, we’ll explore how data and analytics are optimizing pharmaceutical R&D, spotlighting the enormous potential these advanced tools have in reshaping one of the world’s most critical industries.
1. Harnessing Data for Early-Stage Drug Discovery
The first and arguably most crucial stage of pharmaceutical R&D involves identifying viable drug candidates that can target specific diseases or health conditions. Historically, this process was hit-or-miss, relying on trial-and-error experimentation. Today, data analytics has changed the game. By utilizing vast genomic databases and sophisticated computational models, researchers quickly pinpoint molecular structures with a high probability of success. This data-driven approach not only saves time but also drastically reduces costs associated with conventional laboratory tests.
One compelling example lies in the rapid development of therapies for rare diseases. Because such conditions often affect smaller populations, traditional R&D routes can be prohibitively expensive. Data analytics, however, enables scientists to sift through millions of data points—genetic sequences, clinical trial results, and real-world evidence—to identify patterns that might be missed by simple lab work. By zeroing in on the right candidates from the start, pharmaceutical companies can speed up the race to find effective treatments.
It’s not just about discovering new compounds. Many researchers are now repurposing existing drugs by comparing data sets that indicate alternate uses. In other words, data-driven insights maximize the potential of already approved drugs, eliminating the need to start from scratch. This approach is especially beneficial when time is of the essence, such as during global health emergencies. To learn more about how early-stage analytics impacts patient outcomes, consider exploring the resources at IQVIA, which provides a wealth of information on data-enabled innovation in healthcare.
2. Optimizing Clinical Trials Through Advanced Analytics
The clinical trial phase is often described as the most time-consuming and resource-intensive part of the pharmaceutical R&D process. It’s also where data-based decision-making can have a massive impact. By optimizing patient recruitment strategies and real-time monitoring, analytics platforms help researchers identify the right patient cohort and reduce the risk of trial failure.
Real-world examples illustrate how powerful this can be. A large pharmaceutical firm might face lengthy delays because enrolled patients drop out or fail to meet specific criteria. Advanced data analytics can predict which locations are most likely to yield sustained trial participation based on factors like population health data, compliance rates, and healthcare infrastructure. Additionally, ongoing analytics during trials detect anomalies, enabling immediate course corrections. If adverse effects appear in a subset of patients, algorithms can flag these issues early, allowing medical teams to adapt dosages or refine study protocols.
Moreover, wearable devices and digital health apps have opened new doors for continuous patient monitoring. Instead of depending solely on clinic visits, data flows in from patients’ day-to-day activities, painting a more accurate picture of a drug’s effectiveness. This accelerates insights, leading to potentially faster approvals and better patient outcomes. For further discussion on harnessing analytical tools for clinical trials, you can look into case studies from the U.S. Food and Drug Administration (FDA), which actively encourages the integration of real-world data in regulatory decisions.
3. Streamlining Regulatory Approvals and Compliance
Meeting rigorous regulatory requirements can be a daunting task. However, pharmaceutical data analytics can mitigate much of the complexity by automating compliance checks and validating critical documentation. Instead of manually combing through thousands of pages, regulatory teams are using machine learning algorithms that swiftly cross-reference documents against relevant guidelines. This not only expedites approvals but also lessens the chance of human error.
Transparency is another key benefit. Regulators, whether in the U.S. or abroad, often look for clear evidence that a new drug is safe and effective. With data analytics, pharmaceutical firms can generate detailed audit trails that demonstrate adherence to good manufacturing practices (GMP) and clinical standards. The availability of precise data—ranging from manufacturing batch records to electronic health records—strengthens the case for approval. In many instances, shared dashboards allow regulators to track ongoing studies in near real time, promoting collaboration and trust between all stakeholders.
Looking beyond approvals, the post-market surveillance of drugs is critical for detecting any rare but serious side effects. Analytics tools can process vast quantities of patient feedback and adverse event reports, flagging emerging trends before they escalate. As a result, companies proactively mitigate risks and adjust labeling or usage recommendations accordingly. This data-centric approach fosters a safer environment for everyone, helping pharmaceutical organizations maintain their reputation and enhance patient well-being. Internal teams can even use the insights gleaned for further R&D improvements, setting the stage for ongoing innovation.
4. The Future of Pharmaceutical Data Analytics: Personalized Medicine & Beyond
If early discovery and clinical trials are about finding what works on average, the future of analytics in pharmaceuticals aims to discover what works best for each individual. Personalized or precision medicine is rapidly gaining traction as a major healthcare trend. Advances in genetic sequencing and bioinformatics allow researchers to tailor treatments to a patient’s unique genetic makeup, offering a new level of efficacy and reducing adverse reactions. Analytics is the backbone of this movement, converting raw genomic data into actionable insights that lead to individualized therapies.
One exciting possibility is the integration of artificial intelligence (AI) and machine learning to constantly refine drug regimens in real time. Imagine a world where a patient’s smartwatch tracks key health indicators, then communicates with an AI platform that adjusts medication doses to optimize results. This isn’t science fiction—it’s already happening in pilot programs. Continued growth in wearable technology and connected devices paves the way for high-frequency data collection, feeding these adaptive systems and stretching the boundaries of traditional healthcare models.
Moreover, the benefit of cross-vertical implementations is evident: the same analytics principles that guide R&D in one disease area could transform outcomes in others. Insights gleaned from oncology may inform treatments for autoimmune disorders or cardiovascular conditions. Collaboration is the new competitive edge, and the pharmaceutical industry stands to gain enormously by sharing data and analytical models. These shifts won’t just create better drugs; they’ll foster a more patient-centric and proactive healthcare system for everyone.
Conclusion
Data analytics has become a powerful engine driving innovation in pharmaceutical R&D. From accelerating drug discovery to streamlining clinical trials and ensuring smooth regulatory approvals, analytics plays a pivotal role in modernizing and optimizing the entire drug development journey. By harnessing massive data repositories, advanced AI algorithms, and cutting-edge monitoring tools, pharmaceutical companies can shorten development timelines and vastly improve patient outcomes.
Yet this is only the beginning. As personalized medicine gains momentum and interconnected health devices flood the market, the potential for continuous, data-driven improvements grows exponentially. The future will likely see increased collaboration between researchers, healthcare providers, patients, and tech innovators, all working together to transform healthcare from a reactive system into a proactive one.
Are you ready to embrace the next era of pharmaceutical and healthcare innovation? Join the conversation and share your thoughts on how data-powered analytics could reshape the future of healthcare. We invite you to leave a comment, share your own experiences, and continue exploring how these breakthroughs can create a healthier world for everyone.