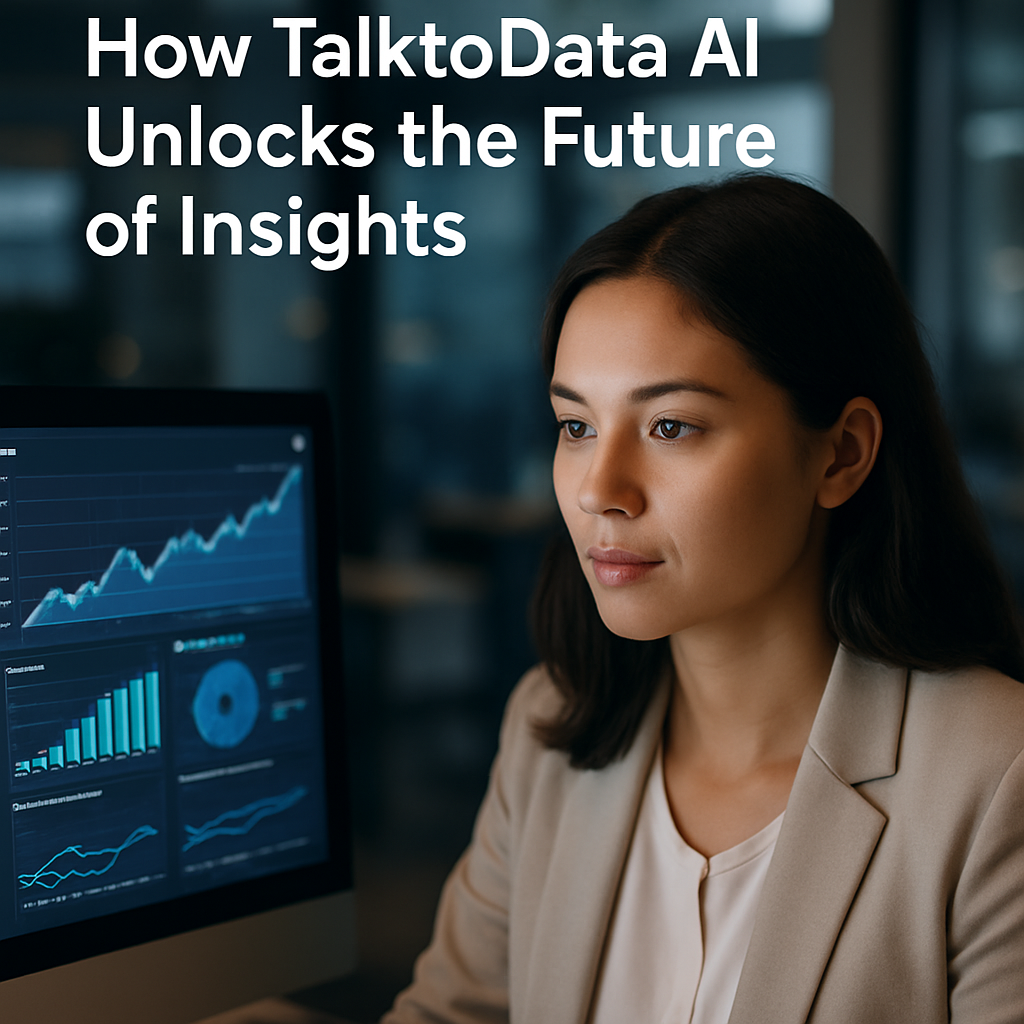
Generative vs. Agentic AI: Choosing the Right Strategy for Your Data Analytics
Generative vs. Agentic AI: Choosing the Right Strategy for Your Data Analytics
Introduction:
In today’s fast-paced digital landscape, businesses are inundated with data. The challenge lies not just in accumulating this data but in extracting actionable insights that drive decision-making. Enter the realm of Artificial Intelligence (AI), a powerful ally in data analytics. Within this domain, two primary types of AI have emerged: generative AI and agentic AI. Each offers unique capabilities and advantages, but how do you choose the right one for your business needs? Join us as we delve into the nuances between these two AI strategies, helping you identify which approach aligns with your data analytics objectives.
Understanding Generative AI
Generative AI refers to algorithms that can generate new content, whether it be text, images, music, or even video, based on the data they have been trained on. This type of AI leverages techniques such as neural networks and deep learning to produce outputs that mimic human creativity. Think of it as a digital artist, capable of creating new masterpieces from existing styles.
For instance, OpenAI's GPT-3 is a prime example of generative AI. It can generate coherent and contextually relevant text, making it invaluable for content creation, marketing, and automated customer interactions. Businesses utilizing generative AI can enhance their customer engagement by producing personalized content at scale, thereby standing out in a crowded marketplace.
The benefits of generative AI include:
- Content Creation: Automating the creation of marketing materials, product descriptions, and even reports.
- Enhanced Creativity: Providing unique ideas and perspectives that might not surface in traditional brainstorming sessions.
- Cost Efficiency: Reducing the time and resources needed for manual content generation.
However, businesses should consider the ethical implications of generative AI, especially regarding copyright issues and the potential for misinformation. Ensuring that the generated content adheres to brand values and legal standards is crucial.
Exploring Agentic AI
Agentic AI, on the other hand, is designed to act autonomously, making decisions based on its environment and the data it processes. Unlike generative AI, which focuses on creation, agentic AI emphasizes interaction and decision-making. Imagine a virtual agent capable of analyzing data patterns and executing strategies in real-time—this is the essence of agentic AI.
A classic example of agentic AI is the use of AI in supply chain management. These systems analyze vast amounts of operational data to optimize inventory levels, forecast demand, and even automate restocking processes. By leveraging agentic AI, businesses can streamline operations, reduce costs, and enhance their responsiveness to market changes.
Key benefits of agentic AI include:
- Real-Time Decision Making: Quickly responding to data changes and market fluctuations.
- Operational Efficiency: Automating repetitive tasks and minimizing human error.
- Predictive Analytics: Using historical data to predict future trends and behaviors.
However, implementing agentic AI requires a careful approach to data governance and security. Ensuring that the algorithms are transparent and accountable is essential in building trust with stakeholders.
Generative AI vs. Agentic AI: Key Differences
While both generative and agentic AI offer significant advantages, understanding their differences is key to choosing the right strategy for your business. Here are some critical distinctions:
- Purpose: Generative AI is primarily concerned with content creation, while agentic AI focuses on decision-making and action.
- Output: Generative AI produces new data, whereas agentic AI analyzes existing data to inform decisions.
- Use Cases: Generative AI finds its place in marketing and creative industries, while agentic AI is more prevalent in operations, logistics, and analytics.
By recognizing these differences, businesses can more effectively align their AI strategies with specific goals and challenges.
Choosing the Right AI Strategy for Your Business
When it comes to integrating AI into your data analytics strategy, the decision between generative and agentic AI should be driven by your business objectives. Start by asking yourself:
- What are the primary goals of my data analytics efforts?
- Do I need to generate unique content or enhance decision-making processes?
- What resources do I have available for implementation and maintenance?
For businesses focused on enhancing marketing efforts and customer engagement, generative AI may be the ideal choice. On the other hand, if the objective is to optimize operations and improve decision-making capabilities, agentic AI would be more suitable. Ultimately, many organizations may find that a hybrid approach, utilizing both types of AI, provides the best results.
Conclusion
In the evolving landscape of data analytics, understanding the distinction between generative and agentic AI is crucial for businesses seeking to leverage technology effectively. Generative AI can foster creativity and streamline content creation, while agentic AI enhances operational efficiency and decision-making capabilities. By carefully considering your unique business needs, you can choose the AI strategy that best supports your objectives. As you navigate this journey, remember that the right AI approach can propel your organization to new heights in data analytics and beyond. What challenges are you facing in your data strategies, and how can AI help you overcome them? Share your thoughts in the comments below!