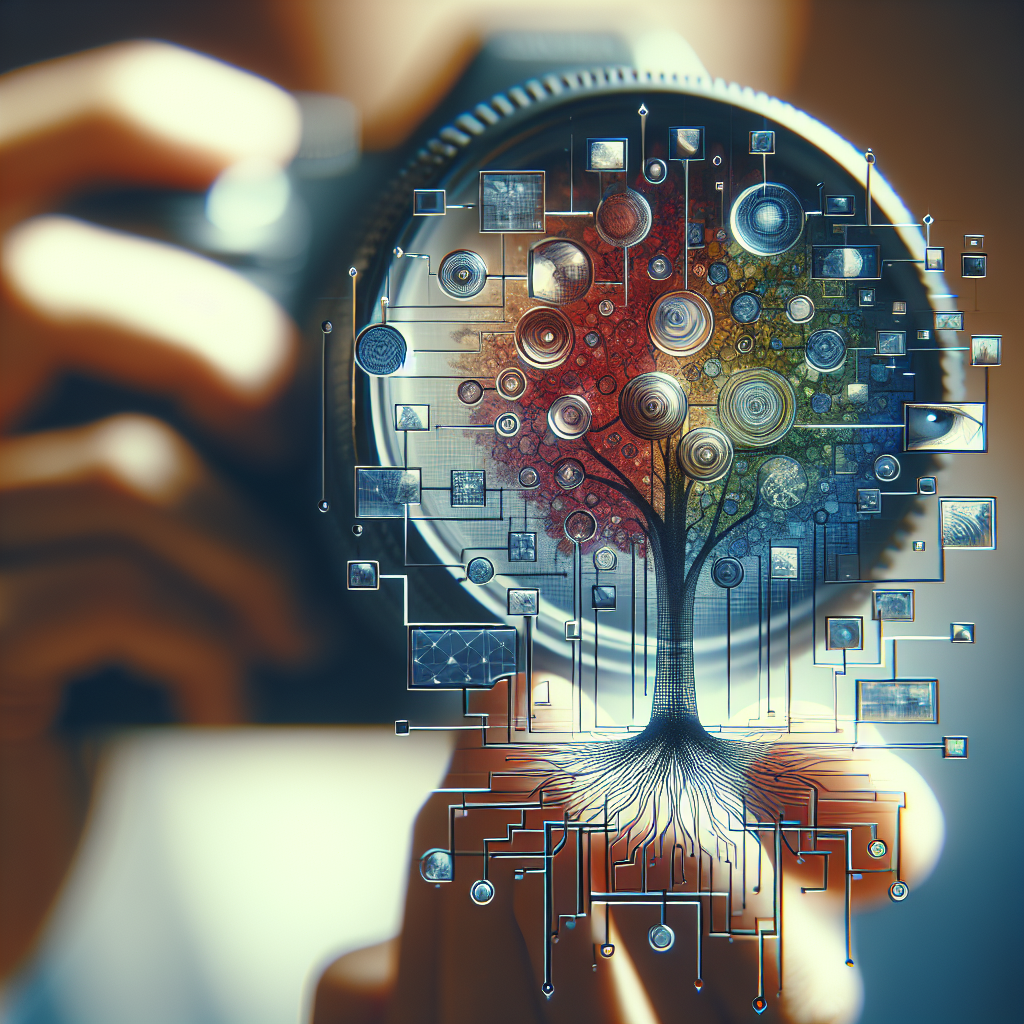
Generative AI vs. Agentic AI: Which is Better for Data Analytics?
Generative AI vs. Agentic AI: Which is Better for Data Analytics?
Introduction:
In today's data-driven world, the terms "Generative AI" and "Agentic AI" are frequently tossed around, often leaving data enthusiasts and business leaders scratching their heads. Both technologies promise to revolutionize the way we analyze data, but they do so in fundamentally different ways. As organizations strive to harness the power of artificial intelligence for data analytics, understanding the distinctions between these two approaches is crucial. This article delves into the nuances of Generative AI and Agentic AI, exploring their roles, effectiveness, and suitability for various analytics applications. By the end, you’ll be equipped to make an informed decision about which AI technology best meets your analytical needs.
Understanding Generative AI
Generative AI refers to algorithms that can generate new content based on the data they are trained on. Think of it as a creative engine that uses existing data to produce unique outputs, whether that's text, images, or even music. For instance, OpenAI's GPT-3 is a prime example of Generative AI, capable of composing essays, generating code snippets, and even drafting poetry. In the realm of data analytics, Generative AI can synthesize insights from large datasets, offering novel interpretations or predicting future trends.
This capability can be particularly beneficial for industries where creativity and innovation are key. For example, in marketing, companies can use Generative AI to create personalized content for their customers based on historical data. Similarly, in product development, it can assist teams in brainstorming new product features by generating ideas from existing customer feedback.
Exploring Agentic AI
Agentic AI, on the other hand, is built with the intention of taking autonomous actions based on data inputs. It acts as an intelligent agent that can analyze real-time data, make decisions, and execute tasks without direct human intervention. A classic example would be recommendation systems used by platforms like Netflix or Amazon, which analyze user behavior to suggest movies or products.
In data analytics, Agentic AI excels in scenarios requiring rapid decision-making and efficiency. For instance, financial institutions utilize Agentic AI to monitor transactions in real-time, flagging potentially fraudulent activities. This proactive approach not only enhances security but also fosters trust among users. In essence, while Generative AI focuses on content creation and insight generation, Agentic AI emphasizes action and implementation.
Comparing Effectiveness in Data Analytics
When it comes to data analytics applications, both Generative and Agentic AI have their strengths and weaknesses. Generative AI is ideal for tasks that require creativity and insight synthesis. It can uncover hidden patterns in data and provide innovative solutions, making it a valuable tool for exploratory data analysis.
In contrast, Agentic AI is better suited for applications that demand quick responses and automation. It can handle large volumes of data with speed and accuracy, allowing businesses to act swiftly on insights. For example, a retail company might employ Agentic AI to optimize inventory management by automatically adjusting stock levels based on real-time sales data.
Ultimately, the choice between Generative AI and Agentic AI hinges on the specific needs of a business. If the goal is to generate new insights or creative solutions, Generative AI would be the way to go. However, if immediate action and efficiency are paramount, Agentic AI emerges as the clear winner.
Real-World Applications of Generative and Agentic AI
To further illustrate the practical implications of these technologies, let’s examine some real-world applications. Companies like Adobe have leveraged Generative AI in their creative software to assist designers in generating unique graphics based on user input. This not only speeds up the design process but also allows for greater creativity.
On the other hand, companies like Google have implemented Agentic AI in their search algorithms. By analyzing user search patterns and behaviors, Google can deliver highly relevant search results in real-time, enhancing user experience. Furthermore, in the automotive industry, companies like Tesla utilize Agentic AI to power their self-driving technology, enabling vehicles to make instantaneous decisions based on environmental data.
These examples underscore the diverse applications of both Generative and Agentic AI across industries, showcasing their unique capabilities and contributions to data analytics.
Conclusion
In conclusion, both Generative AI and Agentic AI offer unique advantages in the realm of data analytics. Generative AI shines in its ability to create and synthesize insights, while Agentic AI excels in executing real-time actions based on data. By understanding the strengths and applications of each technology, businesses can make informed decisions about which AI tools to implement for their specific analytics needs.
So, which AI technology will you choose to enhance your data analytics efforts? Will you opt for the creativity of Generative AI or the efficiency of Agentic AI? We encourage you to share your thoughts and experiences in the comments below, and let’s continue the conversation about the future of AI in data analytics!