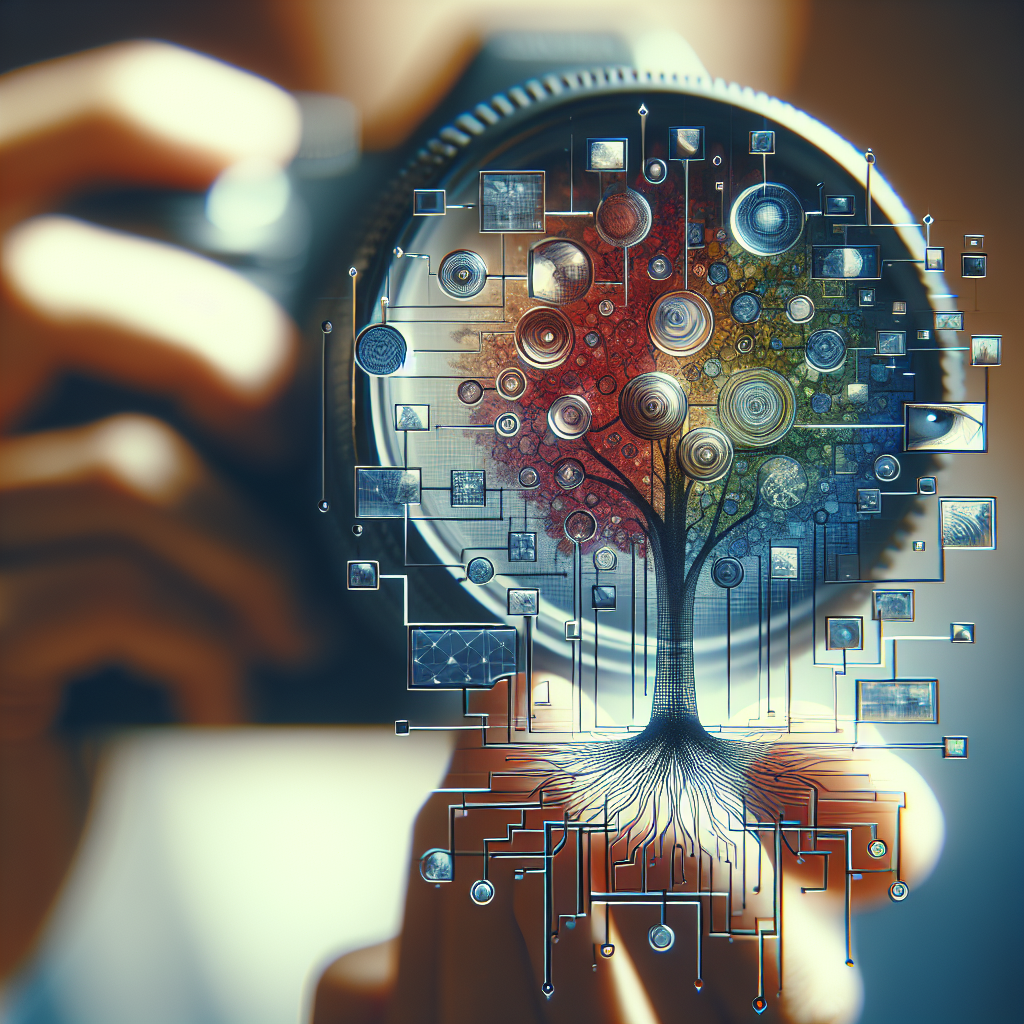
Generative AI vs. Agentic AI: Which is Better for Data Analytics?
Generative AI vs. Agentic AI: Which is Better for Data Analytics?
Introduction:
In the rapidly evolving landscape of artificial intelligence, understanding the differences between Generative AI and Agentic AI is crucial for businesses seeking to leverage data analytics. Both technologies offer unique capabilities, but they serve distinct purposes. This blog post aims to clarify these roles, helping you determine which AI technology aligns best with your specific analytics needs. Are you ready to unlock the potential of AI in your data-driven decision-making? Let’s dive in!
Understanding Generative AI
Generative AI is a type of artificial intelligence that focuses on creating new content based on existing data. Think of it as a digital artist—infusing creativity into data by producing text, images, music, and even sophisticated models. This technology operates on the principles of deep learning and neural networks, analyzing vast datasets to generate outputs that mimic human-like creativity.
For instance, Generative AI can be employed in data analytics to synthesize reports, create visualizations, or even generate predictive models. A well-known example is OpenAI's GPT-3, which produces human-like text and can be used for generating insights from data sets. Businesses can harness the power of Generative AI to enhance storytelling in their reports, making complex analytics more accessible and engaging for stakeholders.
Imagine presenting quarterly sales data not just as numbers, but as an interactive dashboard that narrates the story behind those figures. This capability not only captivates the audience but also drives deeper understanding and engagement with the data.
Exploring Agentic AI
On the other hand, Agentic AI is designed for autonomy and decision-making. It acts as an intelligent agent that can interact with its environment, learning from experiences to make informed decisions. Unlike Generative AI, which focuses on creation, Agentic AI is more about taking decisive actions based on data insights.
Consider a scenario in the world of finance: an Agentic AI system could analyze market trends, execute trades, and even adjust investment strategies in real-time. This technology uses machine learning algorithms to assess risks and opportunities, making it a powerful ally in data analytics for sectors that require quick, data-driven decision-making.
For instance, IBM’s Watson can analyze data from various sources to provide actionable insights for healthcare professionals, helping them make treatment decisions based on real-time patient data. The ability of Agentic AI to adapt and learn from past actions makes it an invaluable tool for businesses aiming to enhance operational efficiency and responsiveness.
Comparing Applications in Data Analytics
When it comes to data analytics, the choice between Generative AI and Agentic AI often depends on the specific needs of the organization. Generative AI shines in scenarios that require creativity and content generation, while Agentic AI excels in decision-making and operational tasks.
For businesses focused on enhancing their data storytelling capabilities, Generative AI is the go-to option. It helps transform raw data into compelling narratives, allowing organizations to communicate insights more effectively. Conversely, if the goal is to automate decision-making processes and improve responsiveness to market changes, then Agentic AI is the better choice.
Moreover, hybrid approaches that combine both technologies can also be explored. For example, a business could use Generative AI to create reports and visualizations while employing Agentic AI to make real-time decisions based on the insights derived from those reports. This integrated approach can lead to more informed strategies and improved outcomes.
Case Studies: Real-World Applications
To truly appreciate the impact of these technologies, let’s explore some real-world applications. In the retail industry, companies like Amazon utilize Generative AI to analyze customer preferences and generate personalized recommendations. This not only enhances the shopping experience but also drives sales through targeted marketing.
On the flip side, companies in the financial sector leverage Agentic AI to manage and analyze vast amounts of transaction data. For instance, JPMorgan Chase uses AI-driven systems to detect fraudulent activities and make instant decisions on whether to flag transactions for review, significantly improving security and efficiency.
These examples underline the distinct benefits that each type of AI brings to the table, demonstrating how organizations can harness their strengths to achieve better data analytics outcomes.
Choosing the Right AI for Your Needs
As businesses navigate the complex landscape of AI technologies, understanding your specific analytics needs is paramount. Start by assessing your objectives: Are you looking to enhance creativity and storytelling through data, or do you need a system that can autonomously make decisions based on real-time insights?
Additionally, consider the integration capabilities of these technologies with your existing systems. A seamless integration can significantly boost the effectiveness of both Generative and Agentic AI in your analytics workflows.
Furthermore, engaging with AI specialists or consulting firms can provide valuable insights into the best practices and strategies for implementing these technologies effectively within your organization.
Conclusion
In the debate of Generative AI versus Agentic AI, there is no one-size-fits-all answer. Each technology offers unique advantages tailored to specific data analytics needs. By understanding the strengths and applications of both, businesses can make informed decisions that enhance their data-driven strategies.
As you embark on your AI journey, reflect on your goals: Are you ready to unlock creative insights with Generative AI, or do you seek the autonomy and decision-making prowess of Agentic AI? We invite you to share your thoughts in the comments below—how do you see AI transforming your data analytics practices? Let’s continue the conversation!