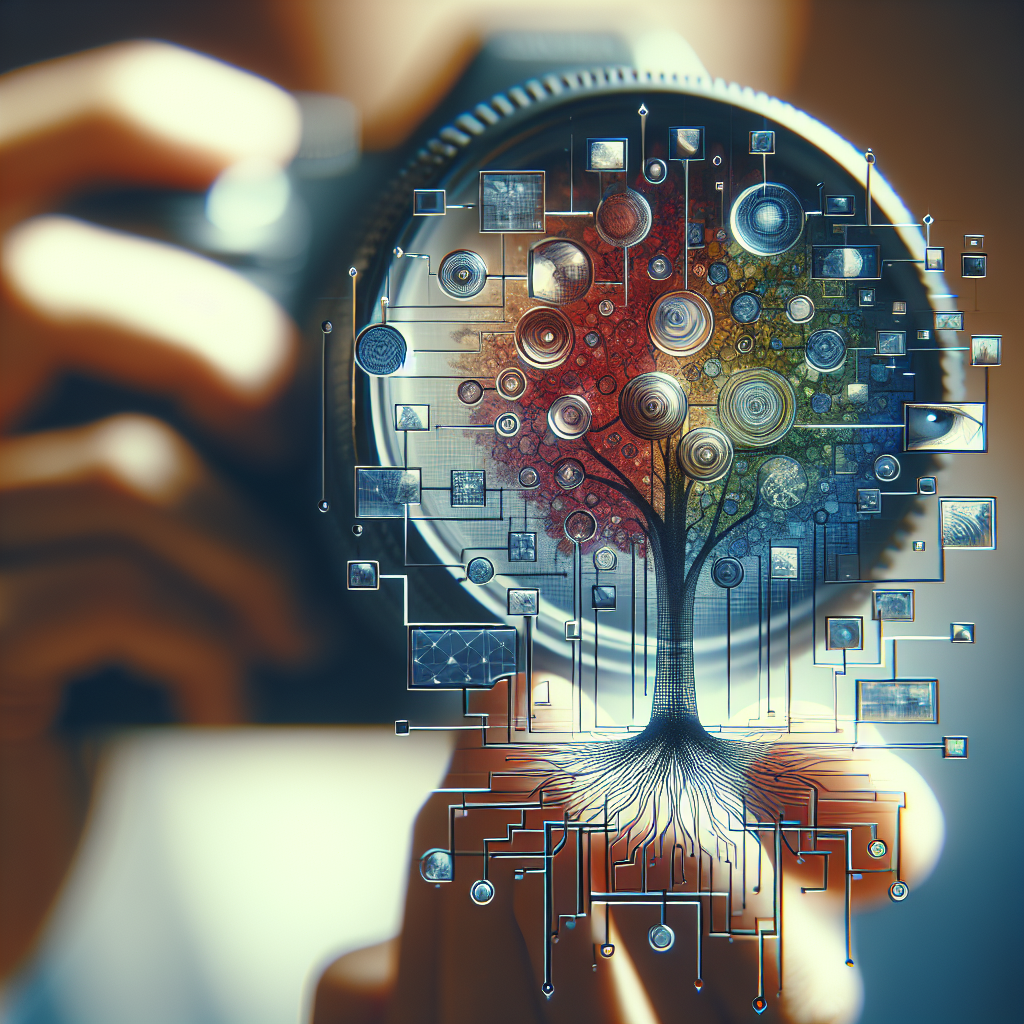
Compliance Considerations for Enterprise AI
Introduction:
Artificial Intelligence (AI) is reshaping how modern enterprises operate, offering new opportunities for growth, efficiency, and innovation. Yet, as organizations become more reliant on AI systems, they also face mounting compliance considerations. Failure to address these considerations can result in legal challenges, reputational risk, and unexpected costs. In this blog post, we’ll delve into the top issues that enterprises should keep in mind when integrating AI solutions into their workflows and discuss strategies for ensuring these systems remain aligned with emerging regulations. Whether you’re a CTO, compliance officer, or business leader, this guide will help you navigate the complex world of enterprise AI without getting caught off guard.
Understanding Regulatory Frameworks for AI
One of the first considerations for enterprise AI initiatives is understanding the broad and evolving range of regulations that govern its use. Around the world, governments and regulatory bodies are increasingly attentive to how companies collect, store, and process data—especially when it involves customer information. For instance, Europe’s General Data Protection Regulation (GDPR) mandates strict rules for data privacy and security, while the U.S. has varying regulations at both state and federal levels, such as the California Consumer Privacy Act (CCPA). Navigating these frameworks calls for expert legal advice, collaboration between IT and compliance teams, and ongoing monitoring of regulatory updates.
Organizations that fail to adapt their AI projects to modern data protection standards could face hefty fines and potential legal actions. A real-world example is the wave of fines imposed by EU regulators on companies found mismanaging user data. Additionally, the increased scrutiny on AI decision-making processes—often referred to as “algorithmic accountability”—means that enterprises must ensure their AI models are transparent, unbiased, and explainable. This is especially critical in highly regulated industries like finance and healthcare, where AI-driven decisions directly affect people’s welfare. By staying proactive and knowledgeable about existing legal requirements, enterprise leaders can align their AI initiatives with acceptable risk and regulatory standards before issues arise.
Ensuring Ethical Data Collection and Usage
Data forms the lifeblood of AI systems, but it also presents significant compliance and ethical considerations. Without careful oversight, an organization could inadvertently harvest personal or proprietary data, incurring legal penalties or damaging its reputation. For example, an enterprise training a recommendation algorithm might rely on consumer behavioral data. If the company fails to inform users or obtain proper consent, it could breach privacy regulations. Moreover, datasets with hidden biases may affect AI outcomes, resulting in unfair or discriminatory results—an outcome no enterprise can afford.
To address these challenges, many organizations are adopting “privacy by design,” an approach that embeds data protection measures throughout the AI development lifecycle. This includes encryption of sensitive information, anonymization procedures, and routine audits to ensure compliance with legal requirements and organizational codes of conduct. In many cases, enterprises also benefit from deploying cross-functional ethics boards to evaluate new AI initiatives. These boards typically involve legal advisors, data scientists, and business stakeholders who collaborate to maintain ethical standards at every stage. By focusing on transparent data practices, companies not only reduce the risk of enforcement actions but also build trust with customers and partners—both of which are vital for achieving long-term operational excellence.
Developing a Robust Governance Model
Another critical compliance component for enterprises implementing AI is establishing a strong governance framework. Governance, in this context, refers to the policies, processes, and structures that guide how AI is developed, deployed, and monitored. It involves defining accountability across different organizational roles, aligning AI initiatives with corporate strategy, and setting clear expectations for performance metrics. For instance, an enterprise that launches an AI-powered fraud detection system needs to determine who evaluates the system’s outputs, how anomalies are handled, and what thresholds trigger human review.
Enterprises often incorporate governance models like the “Three Lines of Defense” approach, separating operational management (first line) from risk oversight (second line) and independent assurance (third line). This structure helps ensure AI projects go through rigorous checks before deployment. In practice, a governance committee might require periodic reports on AI model accuracy, potential bias, and compliance with relevant data protection laws. This level of oversight is particularly useful for AI-driven applications where errors can lead to regulatory scrutiny or financial fallout, such as credit scoring or patented product development. By establishing clear governance protocols, companies can scale their AI efforts responsibly, safeguarding their reputation while delivering measurable business outcomes.
Fostering Continuous Monitoring and Improvement
One of the most overlooked considerations in maintaining AI compliance is the necessity for ongoing monitoring and improvement. AI models evolve over time—either as new data is introduced or as market conditions change. Even a model that was initially in full compliance might drift and produce less reliable or non-compliant results months later. To combat these risks, enterprises should implement performance dashboards and conduct regular model validations. By combining automated alerts with manual audits, an organization can catch potential issues early, whether these arise from data quality problems, regulatory updates, or evolving stakeholder expectations.
In addition, continuous improvement requires an investment in training and upskilling teams across the organization. Data scientists, compliance officers, and operational staff should stay informed about emerging technologies and changing regulations. Regular workshops, online courses, and cross-team collaborations can foster a culture of compliance awareness. Many successful enterprises also maintain relationships with external compliance specialists or industry groups, ensuring they receive timely insights into best practices. This holistic approach makes it far easier to adapt AI models and maintain ethical standards—both of which are essential for competitive advantage in today’s rapidly evolving business environment.
Conclusion
Compliance isn’t a one-time box to check; it’s an ongoing process that evolves alongside advancements in AI technology and shifting regulatory landscapes. By understanding and addressing key considerations—from regulatory frameworks and ethical data usage to governance models and continuous improvement—enterprises can ensure their AI initiatives are both forward-thinking and compliant. Ultimately, embracing comprehensive compliance strategies not only reduces risk but also builds greater trust with stakeholders and customers.
As you look to integrate AI into more aspects of your business, ask yourself: Are your teams equipped to navigate the complexities of enterprise AI? Feel free to share your thoughts in the comments or reach out if you need additional guidance on building a robust, compliant AI strategy. Remember, the future belongs to enterprises that can innovate boldly—without compromising on safety or ethics.