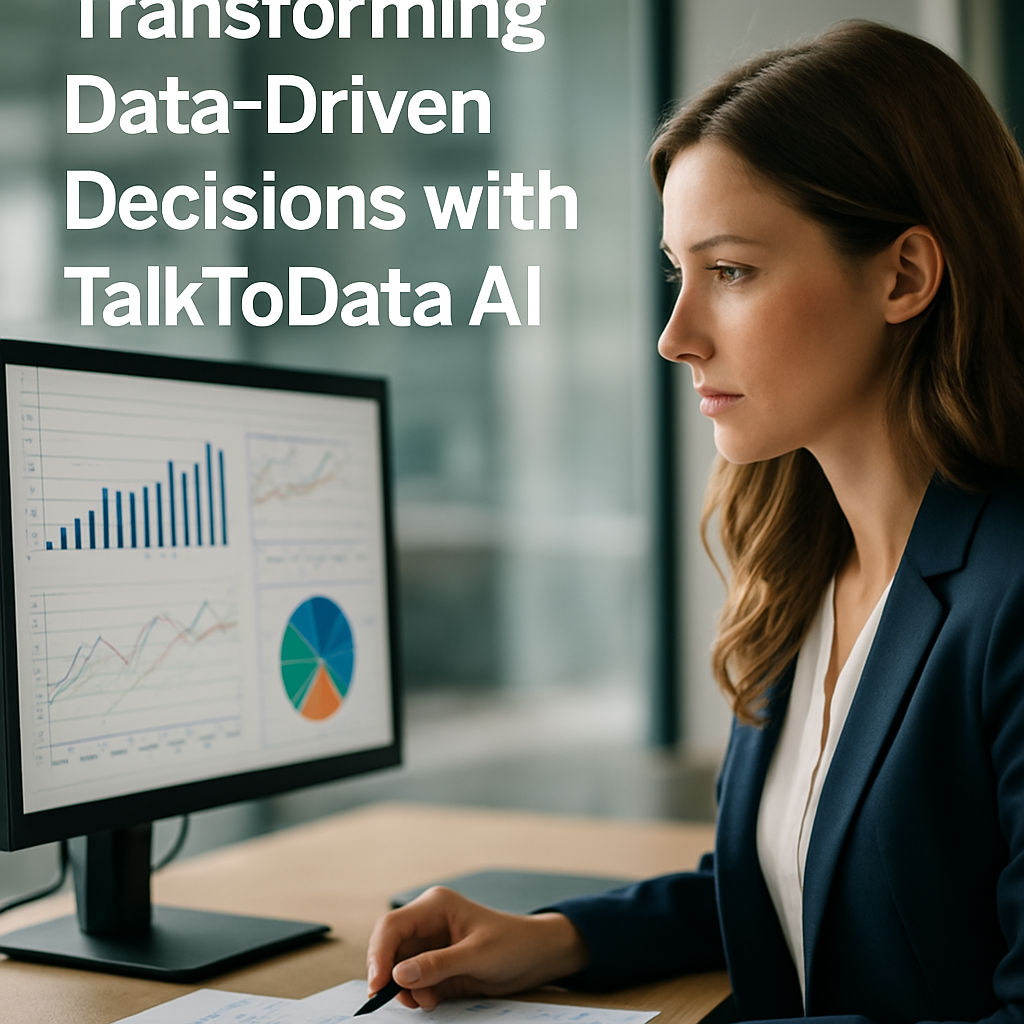
AI-Powered Data Preparation Techniques
Introduction:
Have you ever wondered how businesses manage to turn vast volumes of raw information into meaningful insights? In today’s world, data is the cornerstone of virtually every organizational decision. However, not all data comes in a neat, tidy package. Most datasets require a significant amount of cleanup, structuring, and transformation—collectively known as data preparation. Fortunately, with the advent of AI-powered tools and techniques, this crucial process of organizing and refining raw data has never been more efficient. By leveraging artificial intelligence, companies large and small are saving countless hours otherwise spent on manual data wrangling, drastically improving data accuracy, and enabling teams to focus on interpreting findings rather than grappling with messy records. Whether you’re curious about the latest AI advancements or looking to optimize your analytics workflow, understanding how AI-powered data preparation works is a vital step toward extracting maximum value from your information.
Section 1: The Changing Landscape of Data Preparation
In the early days of data analysis, many organizations relied heavily on spreadsheets, manual coding, or basic statistical tools to organize and refine their raw figures. From filtering out duplicates to concatenating columns, each task required a significant amount of human oversight. As volumes of information soared—especially with the rise of social media, IoT devices, and ecommerce platforms—traditional methods became time-consuming, inflexible, and prone to human error. Imagine a global retailer tasked with consolidating sales, inventory, and customer support logs from dozens of countries. Without automated data preparation techniques, the entire process could spiral into a logistical nightmare.
Enter AI-powered solutions. These groundbreaking tools use machine learning algorithms to detect anomalies, automatically cleanse and format information, and even identify hidden insights during the data ingestion process. For instance, an AI tool might notice that 80% of customer entries in a log file come from a single channel but with slightly different field names. Instead of waiting for a human analyst to spot this discrepancy, AI can suggest a uniform naming convention, standardize the dataset, and flag any outliers for further review. Furthermore, AI-driven analytics can “learn” from each correction made by data engineers, amplifying accuracy over time. This shift has fundamentally changed the data preparation landscape, enabling businesses of all sizes to streamline workflows, reduce errors, and use their manpower more strategically. Organizations can now invest energy into interpreting the refined dataset rather than laboring to diminish duplicates or bridging incompatible data formats. Indeed, the efficiency gained from AI-powered data preparation techniques enables faster, more thorough insights, fueling the modern engine of data-driven decision-making.
Section 2: Key AI-Powered Data Preparation Techniques
One of the most transformative AI-powered data preparation techniques is automated data cleaning. Instead of sifting through extensive datasets to detect invalid entries or missing values, machine learning algorithms identify and rectify these issues instantly. In a typical scenario, a marketing analyst might wrestle with thousands of leads in a CRM system where addresses are missing or incorrectly entered. An AI tool, however, can systematically correct typographical errors and fill in relevant details using information gathered from reliable databases or previous patterns in the dataset. This reduces the time analysts spend unraveling incomplete records and helps maintain accuracy.
Another crucial technique is AI-powered data transformation. Data often arrives in inconsistent formats—for example, dates may appear as “MM/DD/YYYY” in one field and “DD/MM/YYYY” in another. AI tools can rapidly detect these discrepancies and automate uniform transformations to ensure consistency across the dataset. Additionally, natural language processing (NLP) capabilities can parse unstructured text, extracting specific attributes such as sentiment or topic categories. Imagine scanning product reviews and swiftly organizing them by theme or emotional tone—the resulting structured data offers greater clarity for subsequent analysis. Finally, AI tools excel at data enrichment, blending in external datasets to provide a more holistic view. A sales database might be supplemented with regional economic indicators or historical weather data to identify previously unseen correlations. By automating these vital aspects of the preparation workflow, AI-adoption frees professionals from menial tasks, letting them devote time to value-adding processes such as crafting predictive models or refining strategic initiatives.
Section 3: Real-World Applications and Success Stories
Many large enterprises have already reaped the rewards of AI-powered data preparation. Take, for instance, a multinational bank wrestling with client transaction records. Previously, data engineers spent weeks filtering out incomplete or suspicious entries and cross-referencing them against official databases. Now, through machine learning models trained on historical transaction patterns, the bank rapidly flags anomalies and ensures compliance with regulatory standards. The time saved translates directly into streamlined audits and more accurate risk assessments. Another example comes from the healthcare sector, where patient records are scattered across different systems, each using unique classifications for diagnoses and treatments. AI-based platforms efficiently reconcile these discrepancies, providing medical personnel with uniform patient profiles—an innovation that has been pivotal in advancing personalized medicine and ensuring a higher level of care.
In ecommerce, businesses must juggle massive product catalogs sourced from multiple vendors. This yields inconsistencies in naming conventions, descriptions, and even pricing structures. An AI-driven data preparation solution can match these items, deduplicate overlapping sets, and normalize product information in a fraction of the time it would take a manual workforce. As a result, customers enjoy a seamless shopping experience, while the organization benefits from cleaner insights about consumer behavior and opportunities for personalization. Companies that adopt AI-powered data preparation techniques also gain a competitive edge by accelerating their analytics pipelines. Rather than waiting weeks or even months to derive meaningful insights, they can generate near-real-time predictions, enabling agile responses to market changes. It’s no surprise that forward-thinking organizations consider AI-fueled data preparation an essential part of modern business intelligence strategies.
Section 4: Best Practices and Tips for Adopting AI in Data Preparation
Embracing AI-powered data preparation requires a strategic and methodical approach. First, identify the specific pain points in your existing workflow. Are you losing valuable time trying to merge data from disparate systems? Or does your team frequently handle incomplete or messy records? Pinpointing these bottlenecks will guide you toward the most relevant AI solutions. Next, establish clear data governance policies. Even the most advanced AI tools are only as good as the datasets they train on, so standardizing how information is collected, labeled, and updated is paramount. This step also helps maintain compliance with legal and ethical guidelines regarding data handling.
When launching AI initiatives, start small and iterate quickly. Conduct limited proof-of-concept projects that measure the impact of AI-driven techniques on data preparation accuracy and efficiency. If the results are promising, scale up gradually and keep an eye on how the AI model evolves over time. You’ll want to have dedicated oversight to refine algorithms, identify edge cases, and address unanticipated outcomes. Finally, invest in people as much as in technology. Training your team on new tools and methodologies ensures everyone from data analysts to IT personnel can maximize AI advantages. For more advanced tips on AI-enhanced data analysis, you can explore our internal resources in the analytics section of our website. Externally, organizations such as IBM provide thought leadership on effective AI adoption strategies. By combining sound data governance, targeted proof-of-concept pilot programs, and continuous learning, businesses can harness the full power of AI-driven data preparation techniques and transform their decision-making processes.
Conclusion
AI-powered data preparation is rapidly becoming an indispensable component of modern analytics. By automating tasks once handled manually, AI-driven platforms deliver cleaner, more complete datasets in record time. Whether identifying anomalies, unifying formats, or seamlessly integrating external data sources, these solutions empower teams to dive into meaningful analysis without the overhead of scrubbing through countless rows of inconsistent information. As AI models learn from user interventions, their effectiveness grows, further reinforcing the value they offer to organizations of every size and industry. If you’re looking to advance your data analytics strategy, consider experimenting with AI-based systems to streamline and scale your data processing. You’ll not only save time and resources but also unlock a deeper level of insight. Ready to get started? Share your experiences or questions in the comments, and let’s continue this dynamic conversation about AI-powered data preparation techniques.