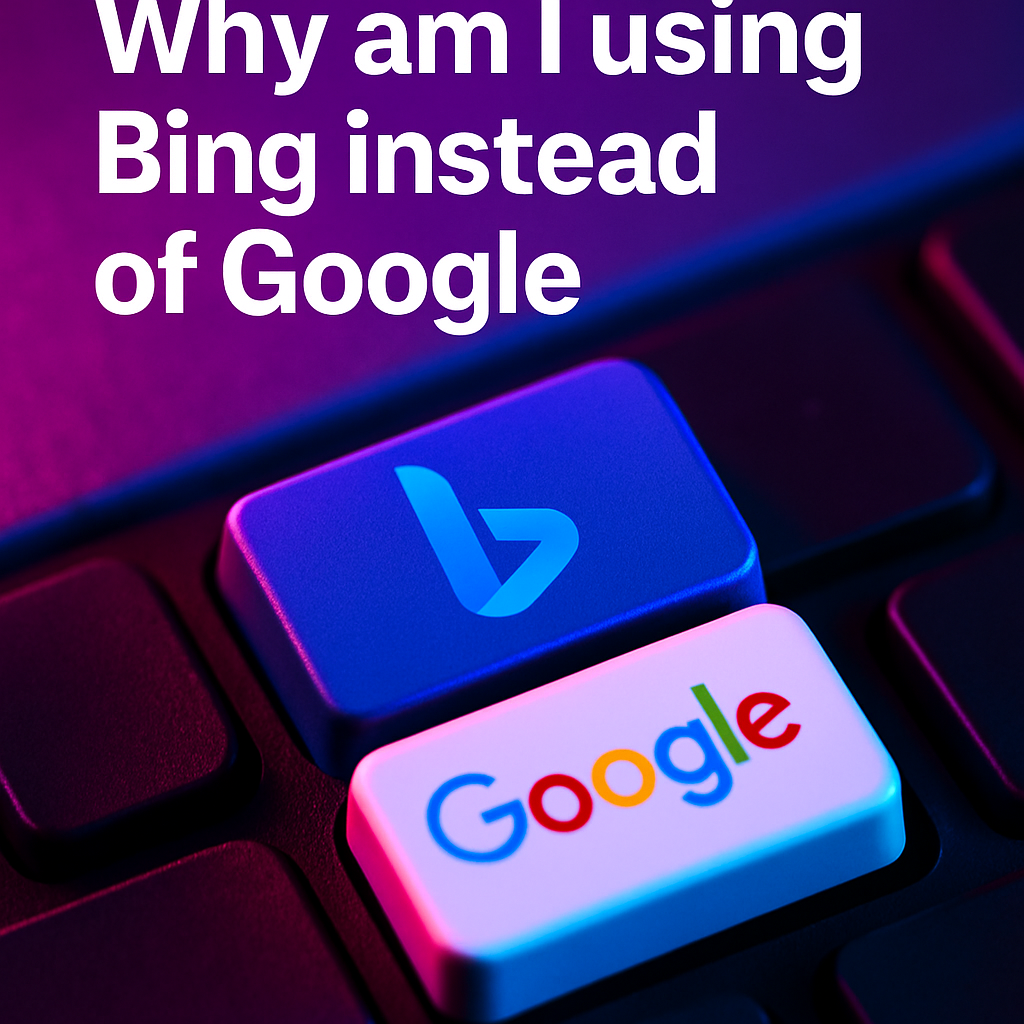
Advanced Statistical Tools in Data Analysis
Introduction:
Are you curious about how businesses, researchers, and analysts unravel hidden patterns in data? Advanced statistical tools are often the secret weapon behind making sense of vast amounts of information. Throughout history, humans have kept records and tracked data, but it’s only in recent decades that technology has empowered us to dig deeper into the numbers. Now, we can employ specialized techniques and sophisticated software to uncover patterns, correlations, and emerging trends. Mastering these advanced strategies not only gives professionals a powerful edge but also ensures that critical decisions are backed by sound logic and accurate insights. In this blog post, we’ll explore how advanced statistical methods can revolutionize your data analysis, offering a glimpse into tools like regression techniques, cluster analysis, predictive modeling, and more. Whether you’re an experienced data analyst, a curious entrepreneur, or a student exploring the endless possibilities of data analysis, these advanced methods will illuminate your path toward better, data-driven results.
Section 1: Understanding the Foundations of Advanced Statistical Analysis
Before diving into more complex tools like structural equation modeling or Bayesian analysis, it’s essential to have a strong foundation in statistics. You might ask, “Why is a firm grasp of the basics critical?” Imagine trying to build a skyscraper without a sturdy base—eventually, it’s bound to topple. The same principle applies to data analysis. Fundamental concepts like probability distributions, sample size determination, and hypothesis testing serve as the groundwork on which you can build more advanced analyses. By revisiting these core ideas, we establish a secure framework to ensure that our advanced methods are both reliable and accurate.
One foundational concept that frequently appears in data analysis is probability. Understanding probability distributions, such as the normal distribution or the Poisson distribution, can help predict how data points are likely to behave. This knowledge, paired with a solid comprehension of measures like mean, median, and standard deviation, allows analysts to quickly understand the nature of a dataset. Additionally, a clear sense of statistical significance—through hypothesis testing—helps analysts determine whether observed effects or patterns are likely to be genuine rather than simply occurring by chance. For instance, while exploring the effectiveness of a brand new marketing campaign, hypothesis testing can reveal if a sudden spike in sales is statistically relevant or just a random fluctuation. By actively applying these foundational elements, you’ll be a step closer to harnessing advanced analytical tools effectively.
Section 2: Exploring Regression Techniques for Deeper Insights
Regression analysis is often considered the backbone of advanced statistical methods. It’s a powerful way to see how one variable may influence another, helping professionals in fields such as finance, healthcare, and marketing interpret data trends. You might be familiar with linear regression. However, regression goes far beyond this simple technique. Multiple regression, polynomial regression, and logarithmic regression each introduce new layers of complexity that can cater to diverse data structures. For instance, multiple regression might be used to investigate how various marketing touchpoints—like email campaigns, social media ads, and event sponsorship—impact a company’s overall revenue.
A practical example involves forecasting seasonal sales. Let’s say a retailer needs to gauge how temperature fluctuations, marketing budgets, and competition collectively affect their monthly voter turnout for loyalty programs. By leveraging multiple regression, analysts can quantify and isolate the effect of each factor, leading to targeted recommendations that can boost membership and engagement. This approach is not just numbers on a page; it leads to actionable strategies that can shape business decisions. Effective analysis guides teams to allocate resources where they will have the greatest impact, fine-tune marketing messages, and forecast demands more accurately. As you continue to refine your statistical abilities, don’t forget to experiment with variants like logistic regression, which is pivotal for binary outcome data (e.g., whether customers will buy a product or not). Understanding when to use these advanced interpretations of regression is key to achieving more robust and more meaningful data analysis.
Section 3: Application of Cluster Analysis and Segmentation Tools
What if you need to group objects, customers, or events based on similarities without a predefined label? That’s where cluster analysis shines. Cluster analysis is an advanced statistical tool that sorts data into naturally occurring groups or “clusters.” This technique comes in handy in numerous scenarios, such as customer segmentation, recommender systems, and even genetic research. For instance, a bank might use cluster analysis to identify groups of customers who share similar financial behaviors. By segmenting them into clusters—say, cautious savers vs. spontaneous spenders—the bank can then tailor specific products or services to fit each group’s distinct needs.
One of the most common cluster analysis techniques is k-means clustering, where analysts predetermine the number of clusters (k) and let the algorithm group data points accordingly. Another method, hierarchical clustering, builds a tree-like structure where the dataset is successively divided into smaller groups, allowing analysts to decide the level of granularity they prefer. Such segmentation tools not only enhance the precision of your analysis but also improve communication among stakeholders. By having clearly identified categories or unique groups, data insights can be communicated more effectively, promoting better strategic decisions. Think of it as color-coding a massive library of books: once everything is organized by subjects that resonate with each other, it’s much easier to locate, understand, and apply the knowledge within. To learn more, you can explore external resources like research papers or specialized tutorials that delve into different clustering algorithms, further broadening your horizons in advanced data analysis.
Section 4: Predictive Modeling and the Future of Data Analysis
Predictive modeling stands at the cutting edge of advanced statistical tools and data analysis, helping organizations stay one step ahead in an ever-changing landscape. These models use historical data to forecast future outcomes, whether it’s the likelihood of a customer purchase, the effectiveness of a new product launch, or the risks associated with policy changes. Techniques like time series forecasting, decision trees, and machine learning models (e.g., random forests or gradient boosting) are now widely accessible through various software packages and programming languages. This accessibility paves the way for companies of all sizes to benefit from predictive insights.
Imagine you run an e-commerce store with thousands of products. By using advanced statistical analysis and predictive modeling, you can estimate which items are likely to surge in demand during specific seasons or events. As a result, you can optimize inventory and save costs related to overstocking or understocking. Outside of business, predictive models are also transforming healthcare, climate science, and public policy. For example, hospitals utilize predictive analytics to identify patients at higher risk of complications, ensuring the right interventions occur at the right time. Meanwhile, meteorologists employ sophisticated models to predict storms or heatwaves more accurately, helping communities prepare for extreme weather events. Consequently, the features that make predictive modeling so powerful—accuracy, flexibility, and scalability—also indicate why it remains a cornerstone of today’s most advanced data analysis strategies. If you’d like to explore these methods further, consider checking reputable data science websites or educational platforms that offer in-depth tutorials.
Conclusion
Advanced statistical tools lie at the heart of modern data analysis, enabling us to interpret complex information in ways that drive innovation and informed decision-making. From mastering the fundamental concepts of probability and hypothesis testing to leveraging regression, cluster analysis, and predictive modeling, each strategy offers unique insights that can turn raw data into actionable knowledge. Whether you’re working in marketing, healthcare, finance, or another data-heavy field, these techniques illuminate patterns and relationships beneath the surface, giving you the power to optimize processes and make predictions with greater accuracy.
Now that you’ve glimpsed the incredible potential of advanced statistical tools, what’s your next step? Consider exploring a new method that intrigues you or diving deeper into a familiar one. Engage with fellow analytics enthusiasts to share newfound wisdom or pose questions in online communities or forums. Remember, data analysis is an ever-evolving discipline—staying current means continually experimenting, learning, and growing. Feel free to share your thoughts or questions in the comments, and pass this post along to colleagues who love digging into data. By expanding your toolkit and maintaining a curious mindset, you’ll be well on your way to becoming a leader in the dynamic world of advanced statistical data analysis.