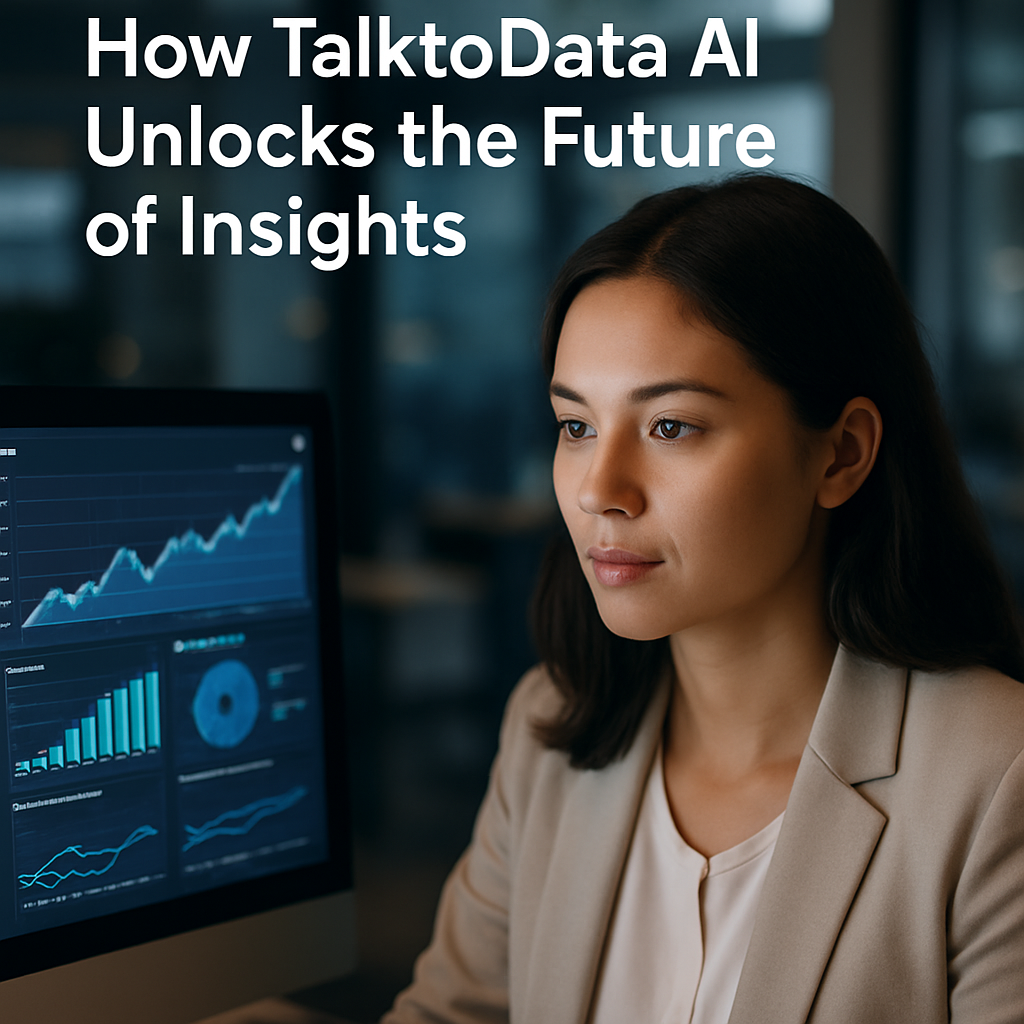
Advanced Applications of Generative AI in Healthcare and Finance
Introduction:
What if machines could learn to create data and insights that go beyond simple predictions? By unlocking the power of Generative AI, organizations across various sectors are increasingly finding ways to revolutionize their practices. In the realms of healthcare and finance, where precision, security, and trust are paramount, these advanced applications of Generative AI hold the potential to reshape both industries in remarkable ways. From streamlining complex drug research and enhancing personalized treatment plans to forecasting risks and diagnosing fraud in real-time, Generative AI has become much more than a theoretical concept—it's an innovative force driving new solutions and strategies.
In this blog post, we dive deep into the advanced applications of Generative AI in Healthcare and Finance, focusing on the intricacies that experienced practitioners value. We’ll explore how cutting-edge techniques like Generative Adversarial Networks (GANs), attention-based Transformers, and advanced language models can be harnessed to tackle sector-specific challenges. Our goal is to provide actionable insights into how these technologies can revolutionize patient care, risk assessment, and decision-making processes. Whether you’re a data scientist, healthcare professional, financial analyst, or simply fascinated by emerging technologies, prepare to discover how generative models are redefining the boundaries of innovation.
1. The Evolution of Generative AI in Healthcare and Finance
Generative AI has come a long way since its inception, evolving from basic pattern recognition systems to sophisticated networks capable of producing synthetic data and accurate predictions. In healthcare, this evolution has been critical for facilitating efficient medical imaging analysis and accelerating drug discovery. For instance, researchers are leveraging Generative Adversarial Networks to synthesize medical images that can augment training datasets for rare disease detection. This additional data helps increase the accuracy of image-based diagnostic tools, ultimately improving patient outcomes.
Meanwhile, in finance, the predictive power of Generative AI has opened doors to more precise market trend analysis and risk assessment. Early adopters used rudimentary machine learning algorithms to produce simple forecasting models. Over time, those models evolved into advanced architectures incorporating neural networks, enabling real-time fraud detection and anomaly recognition. The opportunity to automatically generate vast amounts of synthetic financial scenarios also provides a testing ground for predicting rare market events, helping analysts prepare for unexpected economic turbulence.
Moreover, it’s not just the technology that’s expanding—our understanding of it is, too. As both sectors recognize the inherent value in data-driven decision-making, data scientists and domain experts alike are converging to develop highly specialized models that offer deeper insights. These collaborations have accelerated the adoption of Generative AI by aligning it closely with industry-specific challenges. Consequently, we see a future where healthcare diagnoses become more personalized and financial predictions more robust, transforming both industries from reactive to proactive in their approach.
2. Advanced Techniques Driving Innovation
While traditional AI approaches have relied on supervised learning to make predictions, the latest wave of Generative AI introduces powerful methods such as Generative Adversarial Networks (GANs), diffusion models, and attention-based Transformers. GANs, often described as a two-player game, consist of a generator that produces synthetic data and a discriminator that differentiates between real and synthetic inputs. This adversarial process helps each component improve, leading to data outputs that closely resemble real-world samples. In healthcare, GANs can generate highly realistic X-ray or MRI images to supplement limited datasets, significantly reducing the time required to train diagnostic models. In finance, GANs can simulate market conditions to stress-test trading strategies or verify the robustness of credit-scoring systems.
Diffusion models, a more recent development, excel at complex generation tasks by progressively adding and removing noise from data. This approach helps models capture rich data distributions, making them proficient in tasks like medical image reconstruction, where clarity and detail matter. Meanwhile, attention-based Transformers, such as GPT-style architectures, enable sophisticated natural language processing tasks. Healthcare use cases include summarizing massive volumes of clinical notes for streamlined patient assessments, while in finance, these models assist in generating detailed, data-driven risk analysis reports with minimal human intervention. The ability to process large volumes of unstructured text in real-time is a game-changer, improving efficiency and accuracy.
Crucially, these techniques do more than just offer convenience—they facilitate breakthroughs in how we understand and act on data. By allowing machines to “imagine” scenarios and create new forms of data, practitioners can explore “what-if” conditions to a degree that was once impossible. This innovation leads to safer drug trials, more reliable financial products, and ultimately, a technological edge for organizations willing to invest in these advanced methods.
3. Transformative Impact on Healthcare
Healthcare is experiencing a paradigm shift thanks to the advanced applications of Generative AI. Imagine a world where diagnostic tools not only identify a disease but also propose a personalized treatment plan based on a patient's unique genetic makeup. This is fast becoming a reality. Generative models can create synthetic genomic sequences to help researchers study rare mutations, expediting the search for targeted therapies. Similarly, advanced imaging techniques powered by Generative AI enable doctors to detect subtle anomalies in scans that human eyes might overlook, leading to faster diagnoses and improved patient care.
One of the most promising areas lies in drug discovery. Pharmaceuticals can now harness generative models to predict molecular structures and simulate how these molecules might interact with biological systems. This drastically reduces the time required for preliminary clinical testing, cutting costs and potentially saving lives by accelerating the introduction of new treatments. Patients suffering from chronic and rare conditions stand to benefit immensely, as personalized treatments become the natural extension of these AI-driven breakthroughs. Furthermore, healthcare institutions can use these same generative algorithms to streamline administrative tasks, from automating medical transcriptions to predicting likely readmission rates and optimizing resource allocation.
While challenges such as patient data privacy and regulatory oversight remain significant, there is no denying the remarkable strides being taken. Hospitals are already beginning to rely on AI-assisted triage systems that prioritize high-risk patients, ensuring timely intervention. The convergence of clinical expertise and generative models holds the promise of not only diagnosing conditions more accurately, but also preventing them before they fully manifest, ultimately creating a more proactive health ecosystem.
4. Revolutionizing the Finance Landscape
Finance, often perceived as a numbers game, is finding a new dimension through Generative AI. The days of linear forecasting models and static analytics dashboards are gradually giving way to dynamic, AI-driven risk management and trading solutions. With Generative AI, financial institutions can create lifelike simulations of market behavior, test ventures under various economic scenarios, and even predict chain reactions triggered by global events. This lays the groundwork for more informed decision-making and helps build portfolios that can weather volatile markets.
A compelling example arises in algorithmic trading, where Generative AI can simulate diverse trading conditions, such as sudden market crashes or periods of prolonged bear markets. Traders rely on these simulations to refine algorithms, ensuring that they make data-driven decisions rapidly. Another emerging application is fraud detection. By generating synthetic data representing potential fraudulent activities, banks and payment processors can train their systems to recognize suspicious patterns before they become costly breaches. This forward-thinking approach sharply reduces financial risks and strengthens customer trust.
Additionally, customer-facing solutions benefit from the conversational capabilities of advanced AI models. Chatbots and virtual assistants, armed with large language model technology, can offer real-time financial advice to clients, handle a wide array of account-related inquiries, and even simplify complex processes like loan applications. Such 24/7, on-demand support not only boosts client satisfaction but also frees up human advisors to focus on more intricate tasks that require a personal touch. The capacity to innovate at scale—coupled with robust risk management—illustrates why Generative AI is becoming a critical tool in a rapidly evolving financial landscape.
5. Overcoming Challenges and Ensuring Ethical Implementation
While the benefits of advanced applications of Generative AI in Healthcare and Finance are substantial, challenges still arise around data governance, model interpretability, and regulatory compliance. Healthcare providers must navigate stringent data privacy laws such as HIPAA in the United States and GDPR in the European Union. Patient information is deeply sensitive, so generative models need robust anonymization and encryption protocols to safeguard data. A single breach can undermine trust and create lasting reputational damage, underscoring the importance of reliable security measures.
In the financial sector, compliance with regulations ranging from anti-money laundering (AML) requirements to the Basel Accords adds another layer of complexity. Analysts and AI developers must ensure that the generative models used for market predictions or fraud detection meet stringent accuracy and transparency standards. There is also the challenge of interpretability: Generative AI systems often function as black boxes, making it difficult for stakeholders to understand how certain decisions are reached. In high-stakes fields like healthcare and finance, a lack of transparency can impede adoption and trigger insurance or legal issues.
Ethical considerations extend beyond governance. The potential for biased training data to produce skewed outcomes remains a real concern. This is particularly critical in healthcare, where any bias can adversely affect patient treatment plans. Similarly, in finance, biased models may lead to unfair lending practices. Addressing these issues requires proactive measures such as rigorous auditing, diverse data collection, and continuous monitoring of AI’s outputs. Ultimately, partnering with domain experts to validate AI-driven results can help mitigate risks, ensuring that the technology’s immense benefits are harnessed responsibly and effectively.
Conclusion
Generative AI is far more than a technical marvel—it’s a transformative force reshaping both the healthcare and finance sectors. Through advanced techniques like GANs and attention-based Transformers, these industries are moving toward a future where patient-centric care and data-driven financial decisions become the norm. While challenges around regulations, data privacy, and ethical considerations persist, the proactive and collaborative efforts of researchers, clinicians, financial experts, and AI specialists are paving the way for sustainable, responsible, and innovative applications.
In the coming years, we can expect to see even more sophisticated models capable of rapidly generating new medical discoveries, improving clinical workflows, and forecasting financial markets with unprecedented accuracy. By embracing these breakthroughs, practitioners have the opportunity to elevate standards in both patient care and financial management, making lives healthier and economies more resilient.
Key Takeaways: Generative AI evolves in tandem with real-world demands, enabling better diagnostics, reducing fraud, and shaping future-centric solutions. The potential is immense, but so is the responsibility we carry to implement these technologies ethically and transparently.
Call to Action: Are you already exploring advanced applications of Generative AI in Healthcare and Finance? Share your experiences or questions in the comments below. If this post sparked new ideas, consider sharing it with colleagues or friends who want to stay on the cutting edge of AI-driven innovation.